If you live in Europe you will agree with me that the weather this spring has been the worst since a long time. In fact it was freezing and snowing all through March right into April. And it has not been much better on the East coast in the U.S. We experienced some really strange weather here in Germany, with cold air hovering over the country creating a permanent hazy mist in the air. And a lot of snow everywhere, even at the Easter holiday which normally is green. I have never experienced such conditions before.
But not only was the weather bad. Also the weather forecasts were very unreliable and often downright wrong. For a long time they predicted an imminent arrival of spring – and then it snowed the other day, then they predicted fog and snow when we actually had some sunny, although cold days. Why did this happen?
The answer is – because pattern matching failed. As many do not know, weather forecasts rely heavily on historical data. While meteorlogists will claim that they run complicated simulations on huge supercomputers to predict the weather, in fact most of the predictions are based on experience. Based on a huge collection of data similar meteorological situations of the pasts are searched and matched. Then the historically observed development is extrapolated to the future. More or less in the same way our grandmothers or every farmer is doing it. Don’t get me wrong, this approach still requires enormous computing power and elaborate algorithms, but – it fails if the situation is very unusual. This is what happened this year. Pattern matching failed, because there was no existing pattern to match with. What remains was the pure “model”. But weather is a highly non-linear phenomenon which cannot be predicted by a model, however complex it is. This has been brilliantly shown by nobel prize winner Ilya Prigogine in his book “The end of Certainty”.
We have a similar situation in document understanding. As we know, statistical systems that have been trained by a user (in so-called supervised learning), work well as long as the documents to process somehow match to what has been trained. But beware if there is an unexpected or unknown input. The statistical classifier will in the best case raise a flag, in the worst case produce a false positive result. Because pattern matching needs patterns to match with.
Fortunately human language is not as complex as the weather as it is fairly linear. Therefore it is possible to describe language with a model that can actually describe and understand the text. This is the Semantic Model. With syntactic and semantic analysis of text, software can go beyond pure matching and really understand text as a human does it. Therefore also unknown input can be reliably analyzed and correctly processed. Semantic analysis is the key to understanding text in the future.
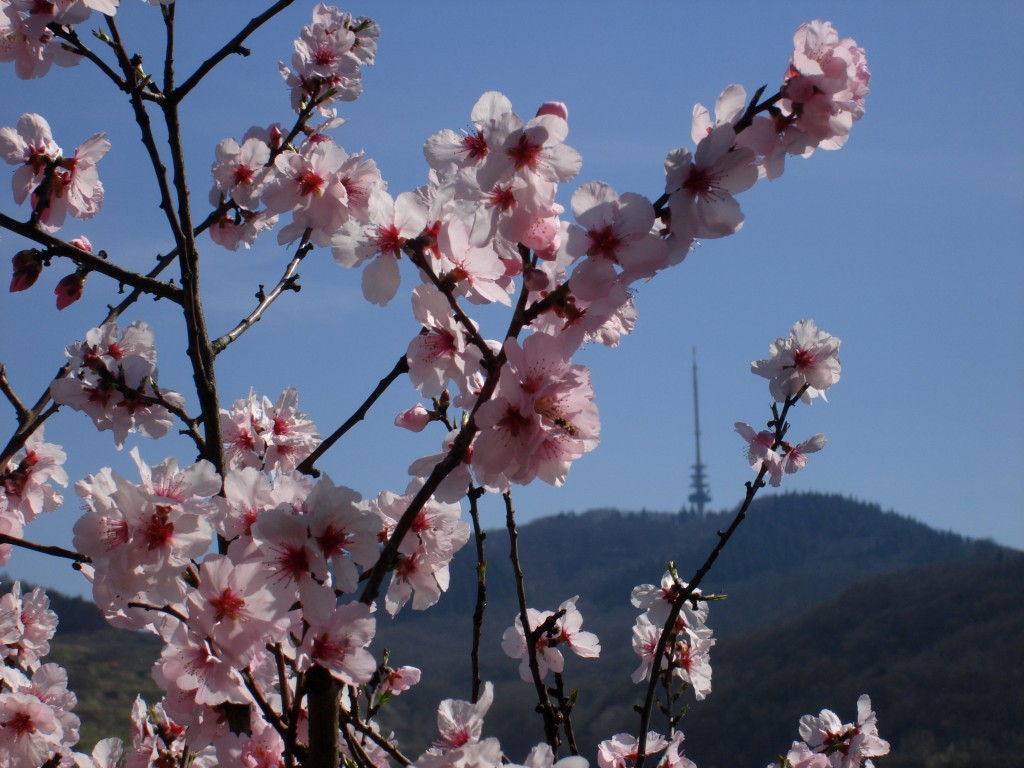
P.S. Finally – as of today – we are back to normal with stable Atlantic west-wind conditions. Forecasts are very precise again and spring has arrived at last. Springtime in Freiburg – in the upper Rhine valley