We all know that our language is fluid and words can change their meaning over time. Words get extinct and new words are created but more often existing words are adapted to new circumstances. It is interesting to see how this happens in the course of years but sometimes words change their meaning overnight.
In a study on “Statistically Significant Detection of Linguistic Change”, published last year (available online from arxiv.org), the researchers Vivek Kulkarni, Rami Al-Rfou, Bryan Perozzi, Steven Skiena have used data mining to find out how the way we use words is revealing the linguistic earthquakes that constantly change our language. There findings are very interesting for anybody who works professionally with text analytics as it reveals a lot about how semantics in our language work. Kulkarni et al. have tracked these linguistic changes by mining the corpus of words stored in databases such as Google Books, movie reviews from Amazon and of course Twitter.
In the pre-internet times the usage and meaning of words changed relatively slowly. This is can be seen in the metamorphoses of the word “gay” from its social meaning in the fifties to the purely sexual-orientation meaning in our time. This is nicely displayed in the word cloud view below:
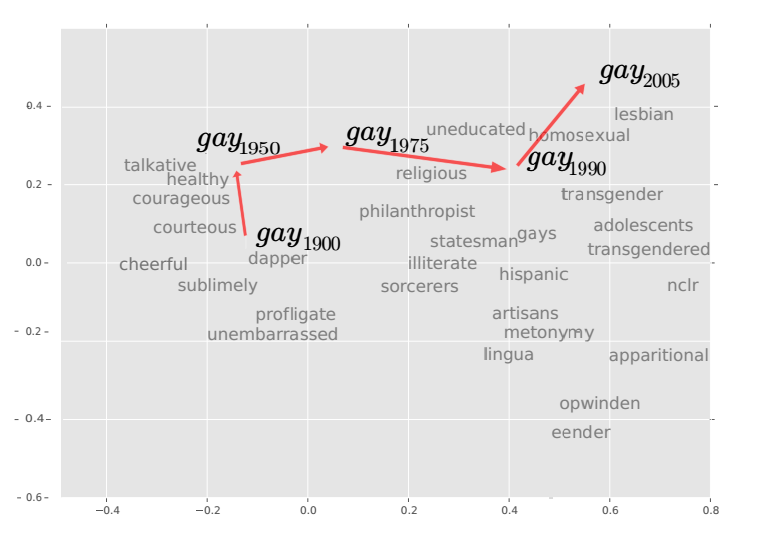
A faster change occurred in the 1970s to the word “mouse”, when it gained the new meaning of “computer input device” and later, the word “windows” was used internationally as the name of the Microsoft operating system within a few years.
Today the meaning of a word can change almost instantly. Before October 2012, the word “sandy” was an adjective meaning “covered in or consisting mostly of sand”. Then Hurricane “Sandy” approached. Almost overnight, this word gained an additional meaning as a proper noun for one of the costliest storms in US history.
Now this might not sound like a big deal for us – but just imagine the insurance industry and the thousands of e-Mails they suddenly receive referring to damages by Sandy! If they are using a static or rule based classification system, they might easily miss the point on these.
So this is a big challenge for automatic classification systems that have been trained in a machine learning algorithm on a specific set of documents containing words in a specific meaning. When these meanings suddenly change or the usage of words is widened suddenly the classifier will make wrong decisions. The only way to cope with this problem in a living and productive system is continuous learning. The system must learn from user corrections – supervised learning – but also from good classification that contains some new aspects and features. This is called unsupervised learning and is so important as the number of documents that can be used for training the system is much higher than the manual correction. By unsupervised learning – or better enhancement, which also includes forgetting btw – the classification system will be able to cope with changed meanings over the time. Abrupt changes as mentioned above will lead to a drop in classification rate from which the classifier will recover within a few days – like humans who will also need a short time to adapt.