Medical Reports are complex documents that are written by doctors who use their specific language and style to express not only facts but also hypotheses and suggestions. They are intended to be read by other doctors or experts who have a deep knowledge of the subject at hand and can make judgements based on what they learn. And in the end the information contained therein is of vital importance for many decisions that are to be taken – medication, change of life style, possible surgery but also cost of insurance.
So reading medical reports using Artificial Intelligence and machine learning is a challenging task. The use case described here utilizes Laera Information Extraction to assist experts at a life insurance to calculate the health risk for persons. In the end the decision needs to be taken by doctors but the system can greatly assist them to sift through the amount of text provided. Because medical reports attached to a life insurance application can easily exceed 100dreds of pages.
Laera Information Extraction uses advanced AI methods to assess the risks contained in these reports and points them out to the experts. In a first step the diagnoses are extracted base on the common ICD-10 code. But a simple word search is not enough because each diagnosis needs to be put into its context. Here the option of Laera to assign multiple roles to an entity becomes very useful. Once a critical diagnosis is found Laera makes an assessment based on several categories:
- Is the polarity negative or positive? In most cases symptoms are excluded in the reports and therefore the diagnosis is negative. These are of no interest (of course the patient is happy about that). Only the positively confirmed ones are relevant for the risk assessment.
- Is the diagnosis for the present or the past? Many reports contain a lot of history of what happened in the past. While the history might be interesting, the main focus is on the current situation.
- Is the diagnosis for the person herself or maybe for the family? Family history (Father had heart attack) might be important but needs to be assessed differently.
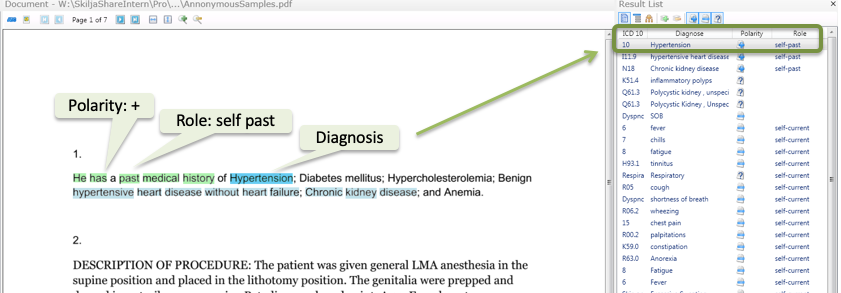
Laera intelligent extraction performs all these tasks and analyzes all pages in milliseconds using semantic and structural methods:
- Find all diagnose and symptoms
- Find the polarity (is the diagnosis excluded or asserted
- Determine the context (role e.g. self or family)
- Classify the paragraph by relevance
- Present and auto-summarize results in ICD10 terms
- Highlight relevant areas in the document for quick visual confirmation
Of course, just to make sure this does not get lost, the roles and assignments are not defined by rules but trained using machine learning from a few hundred labeled examples.
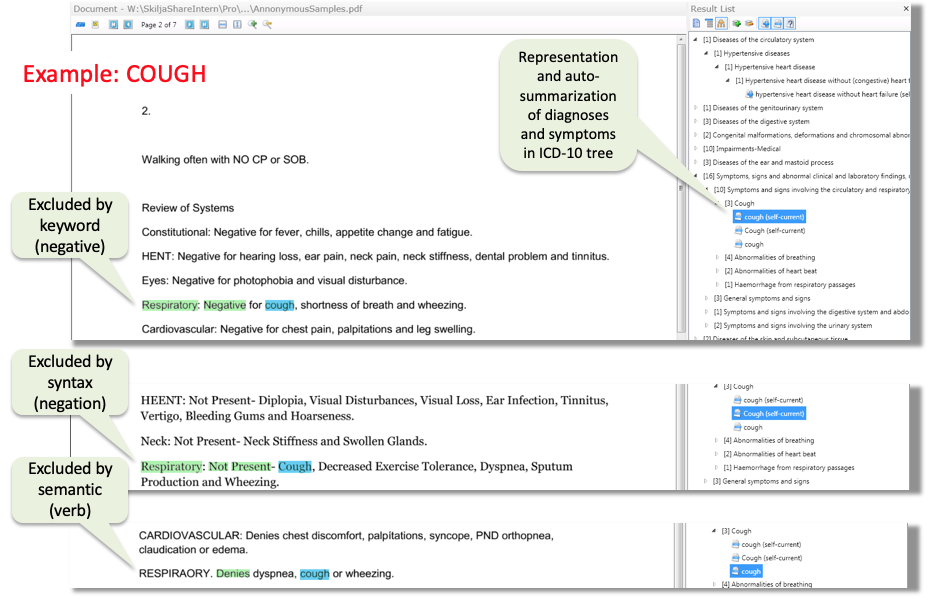
The customer using this system could reduce the time spent to assess an application by more than 50% as the experts get a prepared data set that allows them to quickly jump tp the relevant sections and make the decision.
It is also important to note that this is not a hard coded special solution, but an example of the application of the Laera Information Extraction product. Any other industry can use this approach to solve their specific requirements. Example range from contract management to court documents, but of course also standard default extraction tasks can be easily solved with Laera Information Extraction.
If you are interested to learn more about this use case or the application of AI extraction in your specific domain please let us know via e-Mail at info(at)skilja.com and we will be happy to provide more information.